Edge Detection
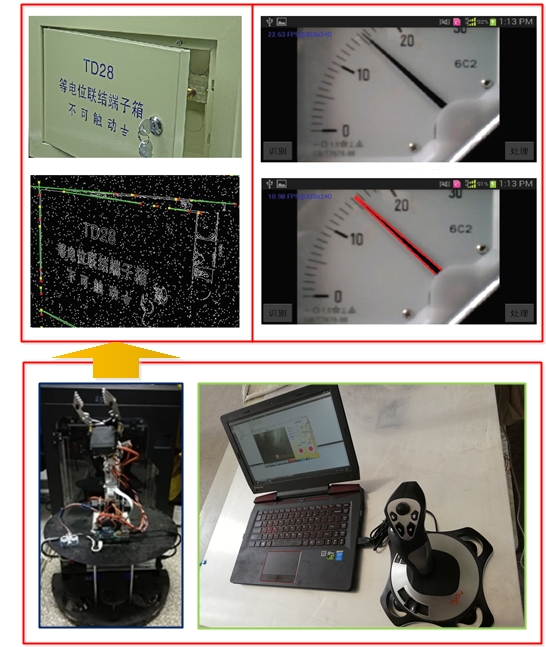
With the increasing intelligent demand of substations, more and more machine vision technologies are applied in smart substations. This project deals with the power cabinet door-opening state recognition based on edge feature extraction.
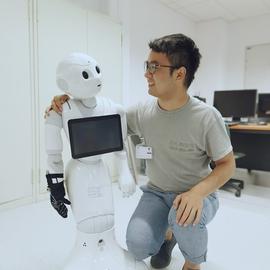
Chuande Liu
Lecturer
My current research interests focus on sensory-based manipulation, robotic motion planning, AI-augmented visual servoing and under-actuated robot systems.
Publications
With the development of the demand for robots to have the ability of opening and unlocking, a real-time detection algorithm for keyhole is proposed in this paper, which combines a fast circle detection based on circle edge feature and a keyhole classifier using self-constructed CNN. The algorithm needs three steps: CNN construction, circle detection and keyhole classification of local circle image. Firstly, collecting keyhole images as positive samples, collecting non-keyhole images and random images as negative samples. Then, constructing a CNN classifier to solve the classification problems and training it based on the samples collected before. Consequently, detecting the real-time collected image whether it has circles and where they are. Furthermore, extract images might have circle area and using the CNN keyhole classifier trained before to determine whether each of them is a keyhole image or not. The experimental results show that using the same training and testing samples, the accuracy of self-constructed CNN classifier(>98 %) is about 1 % lower than that the CNN classifier using AlexNet migration learning network (>99 %), but its time use is about 95% less than the AlexNet and its net size is about 99.5% less than the AlexNet.